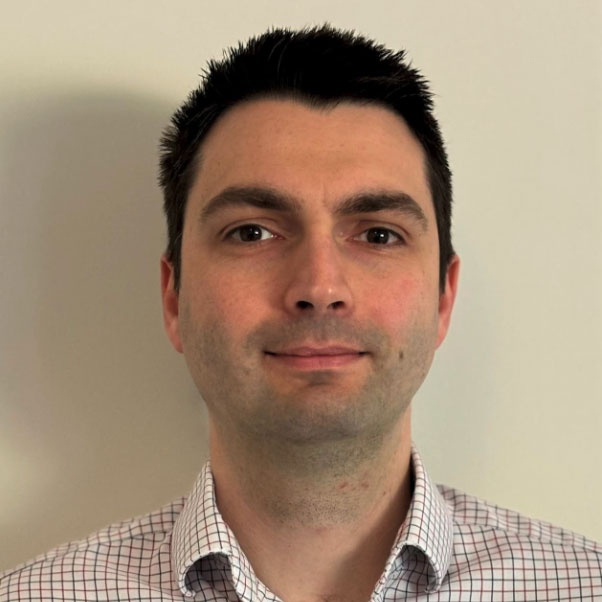
Dr Luke Mcelhinney
Senior Clinical Manager, Medshr
In the hands of a trained specialist, AI may be a game-changer for cardiovascular diseases (CVDs). From predicting heart attacks to enhancing clinical decision-making, learn how AI is reshaping healthcare.
According to the World Health Organization (WHO),1 ‘Prioritising AI for health is crucial.’ What does this mean in practice? Harnessing the vast potential of AI and machine learning, while avoiding their pitfalls, may be a game-changer for CVDs.
Earlier diagnosis of cardiovascular disease
To help predict the risk of a patient developing CVD, the global leading cause of disease and mortality,2 doctors combine their expertise with a variety of tools and algorithms. Recently, a machine-learning CVD risk prediction model was found to have an accuracy of up to 98.4%, outperforming existing tools.3 Such applications of AI could potentially have significant implications for reducing CVD-related morbidity and mortality.
The applications of AI in CVD
diagnosis are promising, with
potential to improve patient outcomes.
AI-assisted interpretation of ECGs and echocardiograms
Echocardiography, cardiac CT and coronary CT angiography (CTCA) — all types of heart scans — are essential tools for diagnosing coronary artery disease (CAD), a form of CVD and a condition that causes heart attacks.
Numerous studies have shown the promise of machine learning in detecting CAD on CTCA and cardiac CT. In one study, a machine learning model accurately interpreted CAD risk based on a finding on cardiac CT scans within six seconds, with similar accuracy to expert interpretation taking 15 minutes.
Interpretation of echocardiography can be subjective, and some signs of CAD are subtle. An AI model recently assessed stress echocardiogram images and could accurately identify patients with severe CAD, helping to inform treatment.
Potential advantages and pitfalls
The applications of AI in CVD diagnosis are promising, with potential to improve patient outcomes; for example, by aiding in detecting, classifying and providing prognosis.6 However, some AI models can be susceptible to generating erroneous information or connections,7 a phenomenon known as a ‘hallucination.’ It is sometimes uncertain how AI arrives at its conclusions.6
Clinicians and healthcare providers who use AI need to be mindful of the potential risks. The WHO emphasised that the use of AI technologies in healthcare should be managed by suitably trained people and that there should be accountability for their use.1
Rather than relying solely on AI, a collaborative approach where clinicians incorporate AI data and recommendations into their decision-making is likely to deliver better outcomes. This was evident in the study on stress echo interpretation, where AI-assisted image interpretation increased clinicians’ performance and confidence in decision-making.5
Looking ahead, the use of AI in CVD diagnosis and other diseases is likely to advance rapidly, guided by further research to maximise benefits while ensuring safety.
[1] WHO, 2021. WHO issues first global report on artificial intelligence (AI) in health and six guiding principles for its design and use. Available:WHO issues first global report on Artificial Intelligence (AI) in health and six guiding principles for its design and use
[2] Sapra V et al, 2023. Integrated Approach using deep neural network and CBR for detecting severity of coronary artery disease. Alexandria Engineering Journal. 68 (1) – 709-720. Available: https://www.sciencedirect.com/science/article/pii/S1110016823000443
[3] Fitriyani N L et al, 2020. HDPM: An effective heart disease prediction model for a clinical decision support system. IEEE Access. Available: https://ieeexplore.ieee.org/stamp/stamp.jsp?arnumber=9144587
[4] Upton R et al, 2022. Automated echocardiographic detection of severe coronary artery disease using artificial intelligence. JACC Cardiovascular Imaging. 15(5): 715-727. https://www.sciencedirect.com/science/article/pii/S1936878X21007804?via%3Dihub
[5] Al-Zaiti S et al, 2023. Machine learning for ECG diagnosis and risk stratification of occlusion myocardial infarction. Nature Medicine. Available: https://www.nature.com/articles/s41591-023-02396-3.pdf
[6] Barry J et al, 2023. The role of artificial intelligence in echocardiography. Journal of Imaging, 9(2), 50. Available: J. Imaging | Free Full-Text | The Role of Artificial Intelligence in Echocardiography (mdpi.com)
[7] Schwartz I S et al, 2024. Black Box Warning: Large language models and the future of infectious diseases consultation. Clinical Infectious Diseases, 78(4): 860-866. Available: Black Box Warning: Large Language Models and the Future of Infectious Diseases Consultation | Clinical Infectious Diseases | Oxford Academic (oup.com)